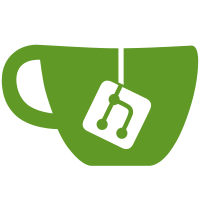
- ``lora_interrogator.py`` is added in ``networks`` folder. See ``python networks\lora_interrogator.py -h`` for usage. - For LoRAs where the activation word is unknown, this script compares the output of Text Encoder after applying LoRA to that of unapplied to find out which token is affected by LoRA. Hopefully you can figure out the activation word. LoRA trained with captions does not seem to be able to interrogate. - Batch size can be large (like 64 or 128). - ``train_textual_inversion.py`` now supports multiple init words. - Following feature is reverted to be the same as before. Sorry for confusion: > Now the number of data in each batch is limited to the number of actual images (not duplicated). Because a certain bucket may contain smaller number of actual images, so the batch may contain same (duplicated) images. - Add new tool to sort, group and average crop image in a dataset
180 lines
6.9 KiB
Python
180 lines
6.9 KiB
Python
|
||
|
||
import argparse
|
||
import os
|
||
import torch
|
||
from safetensors.torch import load_file, save_file
|
||
import library.model_util as model_util
|
||
import lora
|
||
|
||
|
||
def load_state_dict(file_name, dtype):
|
||
if os.path.splitext(file_name)[1] == '.safetensors':
|
||
sd = load_file(file_name)
|
||
else:
|
||
sd = torch.load(file_name, map_location='cpu')
|
||
for key in list(sd.keys()):
|
||
if type(sd[key]) == torch.Tensor:
|
||
sd[key] = sd[key].to(dtype)
|
||
return sd
|
||
|
||
|
||
def save_to_file(file_name, model, state_dict, dtype):
|
||
if dtype is not None:
|
||
for key in list(state_dict.keys()):
|
||
if type(state_dict[key]) == torch.Tensor:
|
||
state_dict[key] = state_dict[key].to(dtype)
|
||
|
||
if os.path.splitext(file_name)[1] == '.safetensors':
|
||
save_file(model, file_name)
|
||
else:
|
||
torch.save(model, file_name)
|
||
|
||
|
||
def merge_to_sd_model(text_encoder, unet, models, ratios, merge_dtype):
|
||
text_encoder.to(merge_dtype)
|
||
unet.to(merge_dtype)
|
||
|
||
# create module map
|
||
name_to_module = {}
|
||
for i, root_module in enumerate([text_encoder, unet]):
|
||
if i == 0:
|
||
prefix = lora.LoRANetwork.LORA_PREFIX_TEXT_ENCODER
|
||
target_replace_modules = lora.LoRANetwork.TEXT_ENCODER_TARGET_REPLACE_MODULE
|
||
else:
|
||
prefix = lora.LoRANetwork.LORA_PREFIX_UNET
|
||
target_replace_modules = lora.LoRANetwork.UNET_TARGET_REPLACE_MODULE
|
||
|
||
for name, module in root_module.named_modules():
|
||
if module.__class__.__name__ in target_replace_modules:
|
||
for child_name, child_module in module.named_modules():
|
||
if child_module.__class__.__name__ == "Linear" or (child_module.__class__.__name__ == "Conv2d" and child_module.kernel_size == (1, 1)):
|
||
lora_name = prefix + '.' + name + '.' + child_name
|
||
lora_name = lora_name.replace('.', '_')
|
||
name_to_module[lora_name] = child_module
|
||
|
||
for model, ratio in zip(models, ratios):
|
||
print(f"loading: {model}")
|
||
lora_sd = load_state_dict(model, merge_dtype)
|
||
|
||
print(f"merging...")
|
||
for key in lora_sd.keys():
|
||
if "lora_down" in key:
|
||
up_key = key.replace("lora_down", "lora_up")
|
||
alpha_key = key[:key.index("lora_down")] + 'alpha'
|
||
|
||
# find original module for this lora
|
||
module_name = '.'.join(key.split('.')[:-2]) # remove trailing ".lora_down.weight"
|
||
if module_name not in name_to_module:
|
||
print(f"no module found for LoRA weight: {key}")
|
||
continue
|
||
module = name_to_module[module_name]
|
||
# print(f"apply {key} to {module}")
|
||
|
||
down_weight = lora_sd[key]
|
||
up_weight = lora_sd[up_key]
|
||
|
||
dim = down_weight.size()[0]
|
||
alpha = lora_sd.get(alpha_key, dim)
|
||
scale = alpha / dim
|
||
|
||
# W <- W + U * D
|
||
weight = module.weight
|
||
if len(weight.size()) == 2:
|
||
# linear
|
||
weight = weight + ratio * (up_weight @ down_weight) * scale
|
||
else:
|
||
# conv2d
|
||
weight = weight + ratio * (up_weight.squeeze(3).squeeze(2) @ down_weight.squeeze(3).squeeze(2)).unsqueeze(2).unsqueeze(3) * scale
|
||
|
||
module.weight = torch.nn.Parameter(weight)
|
||
|
||
|
||
def merge_lora_models(models, ratios, merge_dtype):
|
||
merged_sd = {}
|
||
|
||
alpha = None
|
||
dim = None
|
||
for model, ratio in zip(models, ratios):
|
||
print(f"loading: {model}")
|
||
lora_sd = load_state_dict(model, merge_dtype)
|
||
|
||
print(f"merging...")
|
||
for key in lora_sd.keys():
|
||
if 'alpha' in key:
|
||
if key in merged_sd:
|
||
assert merged_sd[key] == lora_sd[key], f"alpha mismatch / alphaが異なる場合、現時点ではマージできません"
|
||
else:
|
||
alpha = lora_sd[key].detach().numpy()
|
||
merged_sd[key] = lora_sd[key]
|
||
else:
|
||
if key in merged_sd:
|
||
assert merged_sd[key].size() == lora_sd[key].size(
|
||
), f"weights shape mismatch merging v1 and v2, different dims? / 重みのサイズが合いません。v1とv2、または次元数の異なるモデルはマージできません"
|
||
merged_sd[key] = merged_sd[key] + lora_sd[key] * ratio
|
||
else:
|
||
if "lora_down" in key:
|
||
dim = lora_sd[key].size()[0]
|
||
merged_sd[key] = lora_sd[key] * ratio
|
||
|
||
print(f"dim (rank): {dim}, alpha: {alpha}")
|
||
if alpha is None:
|
||
alpha = dim
|
||
|
||
return merged_sd, dim, alpha
|
||
|
||
|
||
def merge(args):
|
||
assert len(args.models) == len(args.ratios), f"number of models must be equal to number of ratios / モデルの数と重みの数は合わせてください"
|
||
|
||
def str_to_dtype(p):
|
||
if p == 'float':
|
||
return torch.float
|
||
if p == 'fp16':
|
||
return torch.float16
|
||
if p == 'bf16':
|
||
return torch.bfloat16
|
||
return None
|
||
|
||
merge_dtype = str_to_dtype(args.precision)
|
||
save_dtype = str_to_dtype(args.save_precision)
|
||
if save_dtype is None:
|
||
save_dtype = merge_dtype
|
||
|
||
if args.sd_model is not None:
|
||
print(f"loading SD model: {args.sd_model}")
|
||
|
||
text_encoder, vae, unet = model_util.load_models_from_stable_diffusion_checkpoint(args.v2, args.sd_model)
|
||
|
||
merge_to_sd_model(text_encoder, unet, args.models, args.ratios, merge_dtype)
|
||
|
||
print(f"saving SD model to: {args.save_to}")
|
||
model_util.save_stable_diffusion_checkpoint(args.v2, args.save_to, text_encoder, unet,
|
||
args.sd_model, 0, 0, save_dtype, vae)
|
||
else:
|
||
state_dict, _, _ = merge_lora_models(args.models, args.ratios, merge_dtype)
|
||
|
||
print(f"saving model to: {args.save_to}")
|
||
save_to_file(args.save_to, state_dict, state_dict, save_dtype)
|
||
|
||
|
||
if __name__ == '__main__':
|
||
parser = argparse.ArgumentParser()
|
||
parser.add_argument("--v2", action='store_true',
|
||
help='load Stable Diffusion v2.x model / Stable Diffusion 2.xのモデルを読み込む')
|
||
parser.add_argument("--save_precision", type=str, default=None,
|
||
choices=[None, "float", "fp16", "bf16"], help="precision in saving, same to merging if omitted / 保存時に精度を変更して保存する、省略時はマージ時の精度と同じ")
|
||
parser.add_argument("--precision", type=str, default="float",
|
||
choices=["float", "fp16", "bf16"], help="precision in merging (float is recommended) / マージの計算時の精度(floatを推奨)")
|
||
parser.add_argument("--sd_model", type=str, default=None,
|
||
help="Stable Diffusion model to load: ckpt or safetensors file, merge LoRA models if omitted / 読み込むモデル、ckptまたはsafetensors。省略時はLoRAモデル同士をマージする")
|
||
parser.add_argument("--save_to", type=str, default=None,
|
||
help="destination file name: ckpt or safetensors file / 保存先のファイル名、ckptまたはsafetensors")
|
||
parser.add_argument("--models", type=str, nargs='*',
|
||
help="LoRA models to merge: ckpt or safetensors file / マージするLoRAモデル、ckptまたはsafetensors")
|
||
parser.add_argument("--ratios", type=float, nargs='*',
|
||
help="ratios for each model / それぞれのLoRAモデルの比率")
|
||
|
||
args = parser.parse_args()
|
||
merge(args)
|