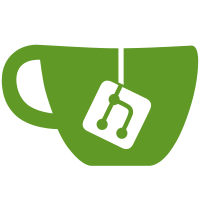
- ``lora_interrogator.py`` is added in ``networks`` folder. See ``python networks\lora_interrogator.py -h`` for usage. - For LoRAs where the activation word is unknown, this script compares the output of Text Encoder after applying LoRA to that of unapplied to find out which token is affected by LoRA. Hopefully you can figure out the activation word. LoRA trained with captions does not seem to be able to interrogate. - Batch size can be large (like 64 or 128). - ``train_textual_inversion.py`` now supports multiple init words. - Following feature is reverted to be the same as before. Sorry for confusion: > Now the number of data in each batch is limited to the number of actual images (not duplicated). Because a certain bucket may contain smaller number of actual images, so the batch may contain same (duplicated) images. - Add new tool to sort, group and average crop image in a dataset
123 lines
5.4 KiB
Python
123 lines
5.4 KiB
Python
|
||
|
||
from tqdm import tqdm
|
||
from library import model_util
|
||
import argparse
|
||
from transformers import CLIPTokenizer
|
||
import torch
|
||
|
||
import library.model_util as model_util
|
||
import lora
|
||
|
||
TOKENIZER_PATH = "openai/clip-vit-large-patch14"
|
||
V2_STABLE_DIFFUSION_PATH = "stabilityai/stable-diffusion-2" # ここからtokenizerだけ使う
|
||
|
||
DEVICE = torch.device('cuda' if torch.cuda.is_available() else 'cpu')
|
||
|
||
|
||
def interrogate(args):
|
||
# いろいろ準備する
|
||
print(f"loading SD model: {args.sd_model}")
|
||
text_encoder, vae, unet = model_util.load_models_from_stable_diffusion_checkpoint(args.v2, args.sd_model)
|
||
|
||
print(f"loading LoRA: {args.model}")
|
||
network = lora.create_network_from_weights(1.0, args.model, vae, text_encoder, unet)
|
||
|
||
# text encoder向けの重みがあるかチェックする:本当はlora側でやるのがいい
|
||
has_te_weight = False
|
||
for key in network.weights_sd.keys():
|
||
if 'lora_te' in key:
|
||
has_te_weight = True
|
||
break
|
||
if not has_te_weight:
|
||
print("This LoRA does not have modules for Text Encoder, cannot interrogate / このLoRAはText Encoder向けのモジュールがないため調査できません")
|
||
return
|
||
del vae
|
||
|
||
print("loading tokenizer")
|
||
if args.v2:
|
||
tokenizer: CLIPTokenizer = CLIPTokenizer.from_pretrained(V2_STABLE_DIFFUSION_PATH, subfolder="tokenizer")
|
||
else:
|
||
tokenizer: CLIPTokenizer = CLIPTokenizer.from_pretrained(TOKENIZER_PATH) # , model_max_length=max_token_length + 2)
|
||
|
||
text_encoder.to(DEVICE)
|
||
text_encoder.eval()
|
||
unet.to(DEVICE)
|
||
unet.eval() # U-Netは呼び出さないので不要だけど
|
||
|
||
# トークンをひとつひとつ当たっていく
|
||
token_id_start = 0
|
||
token_id_end = max(tokenizer.all_special_ids)
|
||
print(f"interrogate tokens are: {token_id_start} to {token_id_end}")
|
||
|
||
def get_all_embeddings(text_encoder):
|
||
embs = []
|
||
with torch.no_grad():
|
||
for token_id in tqdm(range(token_id_start, token_id_end + 1, args.batch_size)):
|
||
batch = []
|
||
for tid in range(token_id, min(token_id_end + 1, token_id + args.batch_size)):
|
||
tokens = [tokenizer.bos_token_id, tid, tokenizer.eos_token_id]
|
||
# tokens = [tid] # こちらは結果がいまひとつ
|
||
batch.append(tokens)
|
||
|
||
# batch_embs = text_encoder(torch.tensor(batch).to(DEVICE))[0].to("cpu") # bos/eosも含めたほうが差が出るようだ [:, 1]
|
||
# clip skip対応
|
||
batch = torch.tensor(batch).to(DEVICE)
|
||
if args.clip_skip is None:
|
||
encoder_hidden_states = text_encoder(batch)[0]
|
||
else:
|
||
enc_out = text_encoder(batch, output_hidden_states=True, return_dict=True)
|
||
encoder_hidden_states = enc_out['hidden_states'][-args.clip_skip]
|
||
encoder_hidden_states = text_encoder.text_model.final_layer_norm(encoder_hidden_states)
|
||
encoder_hidden_states = encoder_hidden_states.to("cpu")
|
||
|
||
embs.extend(encoder_hidden_states)
|
||
return torch.stack(embs)
|
||
|
||
print("get original text encoder embeddings.")
|
||
orig_embs = get_all_embeddings(text_encoder)
|
||
|
||
network.apply_to(text_encoder, unet, True, len(network.unet_loras) > 0)
|
||
network.to(DEVICE)
|
||
network.eval()
|
||
|
||
print("You can ignore warning messages start with '_IncompatibleKeys' (LoRA model does not have alpha because trained by older script) / '_IncompatibleKeys'の警告は無視して構いません(以前のスクリプトで学習されたLoRAモデルのためalphaの定義がありません)")
|
||
print("get text encoder embeddings with lora.")
|
||
lora_embs = get_all_embeddings(text_encoder)
|
||
|
||
# 比べる:とりあえず単純に差分の絶対値で
|
||
print("comparing...")
|
||
diffs = {}
|
||
for i, (orig_emb, lora_emb) in enumerate(zip(orig_embs, tqdm(lora_embs))):
|
||
diff = torch.mean(torch.abs(orig_emb - lora_emb))
|
||
# diff = torch.mean(torch.cosine_similarity(orig_emb, lora_emb, dim=1)) # うまく検出できない
|
||
diff = float(diff.detach().to('cpu').numpy())
|
||
diffs[token_id_start + i] = diff
|
||
|
||
diffs_sorted = sorted(diffs.items(), key=lambda x: -x[1])
|
||
|
||
# 結果を表示する
|
||
print("top 100:")
|
||
for i, (token, diff) in enumerate(diffs_sorted[:100]):
|
||
# if diff < 1e-6:
|
||
# break
|
||
string = tokenizer.convert_tokens_to_string(tokenizer.convert_ids_to_tokens([token]))
|
||
print(f"[{i:3d}]: {token:5d} {string:<20s}: {diff:.5f}")
|
||
|
||
|
||
if __name__ == '__main__':
|
||
parser = argparse.ArgumentParser()
|
||
parser.add_argument("--v2", action='store_true',
|
||
help='load Stable Diffusion v2.x model / Stable Diffusion 2.xのモデルを読み込む')
|
||
parser.add_argument("--sd_model", type=str, default=None,
|
||
help="Stable Diffusion model to load: ckpt or safetensors file / 読み込むSDのモデル、ckptまたはsafetensors")
|
||
parser.add_argument("--model", type=str, default=None,
|
||
help="LoRA model to interrogate: ckpt or safetensors file / 調査するLoRAモデル、ckptまたはsafetensors")
|
||
parser.add_argument("--batch_size", type=int, default=16,
|
||
help="batch size for processing with Text Encoder / Text Encoderで処理するときのバッチサイズ")
|
||
parser.add_argument("--clip_skip", type=int, default=None,
|
||
help="use output of nth layer from back of text encoder (n>=1) / text encoderの後ろからn番目の層の出力を用いる(nは1以上)")
|
||
|
||
args = parser.parse_args()
|
||
interrogate(args)
|