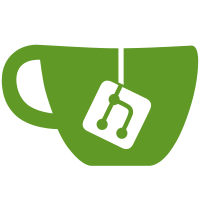
- ``lora_interrogator.py`` is added in ``networks`` folder. See ``python networks\lora_interrogator.py -h`` for usage. - For LoRAs where the activation word is unknown, this script compares the output of Text Encoder after applying LoRA to that of unapplied to find out which token is affected by LoRA. Hopefully you can figure out the activation word. LoRA trained with captions does not seem to be able to interrogate. - Batch size can be large (like 64 or 128). - ``train_textual_inversion.py`` now supports multiple init words. - Following feature is reverted to be the same as before. Sorry for confusion: > Now the number of data in each batch is limited to the number of actual images (not duplicated). Because a certain bucket may contain smaller number of actual images, so the batch may contain same (duplicated) images. - Add new tool to sort, group and average crop image in a dataset
503 lines
22 KiB
Python
503 lines
22 KiB
Python
import importlib
|
||
import argparse
|
||
import gc
|
||
import math
|
||
import os
|
||
|
||
from tqdm import tqdm
|
||
import torch
|
||
from accelerate.utils import set_seed
|
||
import diffusers
|
||
from diffusers import DDPMScheduler
|
||
|
||
import library.train_util as train_util
|
||
from library.train_util import DreamBoothDataset, FineTuningDataset
|
||
|
||
imagenet_templates_small = [
|
||
"a photo of a {}",
|
||
"a rendering of a {}",
|
||
"a cropped photo of the {}",
|
||
"the photo of a {}",
|
||
"a photo of a clean {}",
|
||
"a photo of a dirty {}",
|
||
"a dark photo of the {}",
|
||
"a photo of my {}",
|
||
"a photo of the cool {}",
|
||
"a close-up photo of a {}",
|
||
"a bright photo of the {}",
|
||
"a cropped photo of a {}",
|
||
"a photo of the {}",
|
||
"a good photo of the {}",
|
||
"a photo of one {}",
|
||
"a close-up photo of the {}",
|
||
"a rendition of the {}",
|
||
"a photo of the clean {}",
|
||
"a rendition of a {}",
|
||
"a photo of a nice {}",
|
||
"a good photo of a {}",
|
||
"a photo of the nice {}",
|
||
"a photo of the small {}",
|
||
"a photo of the weird {}",
|
||
"a photo of the large {}",
|
||
"a photo of a cool {}",
|
||
"a photo of a small {}",
|
||
]
|
||
|
||
imagenet_style_templates_small = [
|
||
"a painting in the style of {}",
|
||
"a rendering in the style of {}",
|
||
"a cropped painting in the style of {}",
|
||
"the painting in the style of {}",
|
||
"a clean painting in the style of {}",
|
||
"a dirty painting in the style of {}",
|
||
"a dark painting in the style of {}",
|
||
"a picture in the style of {}",
|
||
"a cool painting in the style of {}",
|
||
"a close-up painting in the style of {}",
|
||
"a bright painting in the style of {}",
|
||
"a cropped painting in the style of {}",
|
||
"a good painting in the style of {}",
|
||
"a close-up painting in the style of {}",
|
||
"a rendition in the style of {}",
|
||
"a nice painting in the style of {}",
|
||
"a small painting in the style of {}",
|
||
"a weird painting in the style of {}",
|
||
"a large painting in the style of {}",
|
||
]
|
||
|
||
|
||
def collate_fn(examples):
|
||
return examples[0]
|
||
|
||
|
||
def train(args):
|
||
if args.output_name is None:
|
||
args.output_name = args.token_string
|
||
use_template = args.use_object_template or args.use_style_template
|
||
|
||
train_util.verify_training_args(args)
|
||
train_util.prepare_dataset_args(args, True)
|
||
|
||
cache_latents = args.cache_latents
|
||
use_dreambooth_method = args.in_json is None
|
||
|
||
if args.seed is not None:
|
||
set_seed(args.seed)
|
||
|
||
tokenizer = train_util.load_tokenizer(args)
|
||
|
||
# acceleratorを準備する
|
||
print("prepare accelerator")
|
||
accelerator, unwrap_model = train_util.prepare_accelerator(args)
|
||
|
||
# mixed precisionに対応した型を用意しておき適宜castする
|
||
weight_dtype, save_dtype = train_util.prepare_dtype(args)
|
||
|
||
# モデルを読み込む
|
||
text_encoder, vae, unet, _ = train_util.load_target_model(args, weight_dtype)
|
||
|
||
# Convert the init_word to token_id
|
||
if args.init_word is not None:
|
||
init_token_ids = tokenizer.encode(args.init_word, add_special_tokens=False)
|
||
if len(init_token_ids) > 1 and len(init_token_ids) != args.num_vectors_per_token:
|
||
print(
|
||
f"token length for init words is not same to num_vectors_per_token, init words is repeated or truncated / 初期化単語のトークン長がnum_vectors_per_tokenと合わないため、繰り返しまたは切り捨てが発生します: length {len(init_token_ids)}")
|
||
else:
|
||
init_token_ids = None
|
||
|
||
# add new word to tokenizer, count is num_vectors_per_token
|
||
token_strings = [args.token_string] + [f"{args.token_string}{i+1}" for i in range(args.num_vectors_per_token - 1)]
|
||
num_added_tokens = tokenizer.add_tokens(token_strings)
|
||
assert num_added_tokens == args.num_vectors_per_token, f"tokenizer has same word to token string. please use another one / 指定したargs.token_stringは既に存在します。別の単語を使ってください: {args.token_string}"
|
||
|
||
token_ids = tokenizer.convert_tokens_to_ids(token_strings)
|
||
print(f"tokens are added: {token_ids}")
|
||
assert min(token_ids) == token_ids[0] and token_ids[-1] == token_ids[0] + len(token_ids) - 1, f"token ids is not ordered"
|
||
assert len(tokenizer) - 1 == token_ids[-1], f"token ids is not end of tokenize: {len(tokenizer)}"
|
||
|
||
# Resize the token embeddings as we are adding new special tokens to the tokenizer
|
||
text_encoder.resize_token_embeddings(len(tokenizer))
|
||
|
||
# Initialise the newly added placeholder token with the embeddings of the initializer token
|
||
token_embeds = text_encoder.get_input_embeddings().weight.data
|
||
if init_token_ids is not None:
|
||
for i, token_id in enumerate(token_ids):
|
||
token_embeds[token_id] = token_embeds[init_token_ids[i % len(init_token_ids)]]
|
||
# print(token_id, token_embeds[token_id].mean(), token_embeds[token_id].min())
|
||
|
||
# load weights
|
||
if args.weights is not None:
|
||
embeddings = load_weights(args.weights)
|
||
assert len(token_ids) == len(
|
||
embeddings), f"num_vectors_per_token is mismatch for weights / 指定した重みとnum_vectors_per_tokenの値が異なります: {len(embeddings)}"
|
||
# print(token_ids, embeddings.size())
|
||
for token_id, embedding in zip(token_ids, embeddings):
|
||
token_embeds[token_id] = embedding
|
||
# print(token_id, token_embeds[token_id].mean(), token_embeds[token_id].min())
|
||
print(f"weighs loaded")
|
||
|
||
print(f"create embeddings for {args.num_vectors_per_token} tokens, for {args.token_string}")
|
||
|
||
# データセットを準備する
|
||
if use_dreambooth_method:
|
||
print("Use DreamBooth method.")
|
||
train_dataset = DreamBoothDataset(args.train_batch_size, args.train_data_dir, args.reg_data_dir,
|
||
tokenizer, args.max_token_length, args.caption_extension, args.shuffle_caption, args.keep_tokens,
|
||
args.resolution, args.enable_bucket, args.min_bucket_reso, args.max_bucket_reso,
|
||
args.bucket_reso_steps, args.bucket_no_upscale,
|
||
args.prior_loss_weight, args.flip_aug, args.color_aug, args.face_crop_aug_range, args.random_crop, args.debug_dataset)
|
||
else:
|
||
print("Train with captions.")
|
||
train_dataset = FineTuningDataset(args.in_json, args.train_batch_size, args.train_data_dir,
|
||
tokenizer, args.max_token_length, args.shuffle_caption, args.keep_tokens,
|
||
args.resolution, args.enable_bucket, args.min_bucket_reso, args.max_bucket_reso,
|
||
args.bucket_reso_steps, args.bucket_no_upscale,
|
||
args.flip_aug, args.color_aug, args.face_crop_aug_range, args.random_crop,
|
||
args.dataset_repeats, args.debug_dataset)
|
||
|
||
# make captions: tokenstring tokenstring1 tokenstring2 ...tokenstringn という文字列に書き換える超乱暴な実装
|
||
if use_template:
|
||
print("use template for training captions. is object: {args.use_object_template}")
|
||
templates = imagenet_templates_small if args.use_object_template else imagenet_style_templates_small
|
||
replace_to = " ".join(token_strings)
|
||
captions = []
|
||
for tmpl in templates:
|
||
captions.append(tmpl.format(replace_to))
|
||
train_dataset.add_replacement("", captions)
|
||
elif args.num_vectors_per_token > 1:
|
||
replace_to = " ".join(token_strings)
|
||
train_dataset.add_replacement(args.token_string, replace_to)
|
||
|
||
train_dataset.make_buckets()
|
||
|
||
if args.debug_dataset:
|
||
train_util.debug_dataset(train_dataset, show_input_ids=True)
|
||
return
|
||
if len(train_dataset) == 0:
|
||
print("No data found. Please verify arguments / 画像がありません。引数指定を確認してください")
|
||
return
|
||
|
||
# モデルに xformers とか memory efficient attention を組み込む
|
||
train_util.replace_unet_modules(unet, args.mem_eff_attn, args.xformers)
|
||
|
||
# 学習を準備する
|
||
if cache_latents:
|
||
vae.to(accelerator.device, dtype=weight_dtype)
|
||
vae.requires_grad_(False)
|
||
vae.eval()
|
||
with torch.no_grad():
|
||
train_dataset.cache_latents(vae)
|
||
vae.to("cpu")
|
||
if torch.cuda.is_available():
|
||
torch.cuda.empty_cache()
|
||
gc.collect()
|
||
|
||
if args.gradient_checkpointing:
|
||
unet.enable_gradient_checkpointing()
|
||
text_encoder.gradient_checkpointing_enable()
|
||
|
||
# 学習に必要なクラスを準備する
|
||
print("prepare optimizer, data loader etc.")
|
||
|
||
# 8-bit Adamを使う
|
||
if args.use_8bit_adam:
|
||
try:
|
||
import bitsandbytes as bnb
|
||
except ImportError:
|
||
raise ImportError("No bitsand bytes / bitsandbytesがインストールされていないようです")
|
||
print("use 8-bit Adam optimizer")
|
||
optimizer_class = bnb.optim.AdamW8bit
|
||
else:
|
||
optimizer_class = torch.optim.AdamW
|
||
|
||
trainable_params = text_encoder.get_input_embeddings().parameters()
|
||
|
||
# betaやweight decayはdiffusers DreamBoothもDreamBooth SDもデフォルト値のようなのでオプションはとりあえず省略
|
||
optimizer = optimizer_class(trainable_params, lr=args.learning_rate)
|
||
|
||
# dataloaderを準備する
|
||
# DataLoaderのプロセス数:0はメインプロセスになる
|
||
n_workers = min(args.max_data_loader_n_workers, os.cpu_count() - 1) # cpu_count-1 ただし最大で指定された数まで
|
||
train_dataloader = torch.utils.data.DataLoader(
|
||
train_dataset, batch_size=1, shuffle=False, collate_fn=collate_fn, num_workers=n_workers, persistent_workers=args.persistent_data_loader_workers)
|
||
|
||
# 学習ステップ数を計算する
|
||
if args.max_train_epochs is not None:
|
||
args.max_train_steps = args.max_train_epochs * len(train_dataloader)
|
||
print(f"override steps. steps for {args.max_train_epochs} epochs is / 指定エポックまでのステップ数: {args.max_train_steps}")
|
||
|
||
# lr schedulerを用意する
|
||
lr_scheduler = diffusers.optimization.get_scheduler(
|
||
args.lr_scheduler, optimizer, num_warmup_steps=args.lr_warmup_steps, num_training_steps=args.max_train_steps * args.gradient_accumulation_steps)
|
||
|
||
# acceleratorがなんかよろしくやってくれるらしい
|
||
text_encoder, optimizer, train_dataloader, lr_scheduler = accelerator.prepare(
|
||
text_encoder, optimizer, train_dataloader, lr_scheduler)
|
||
|
||
index_no_updates = torch.arange(len(tokenizer)) < token_ids[0]
|
||
# print(len(index_no_updates), torch.sum(index_no_updates))
|
||
orig_embeds_params = unwrap_model(text_encoder).get_input_embeddings().weight.data.detach().clone()
|
||
|
||
# Freeze all parameters except for the token embeddings in text encoder
|
||
text_encoder.requires_grad_(True)
|
||
text_encoder.text_model.encoder.requires_grad_(False)
|
||
text_encoder.text_model.final_layer_norm.requires_grad_(False)
|
||
text_encoder.text_model.embeddings.position_embedding.requires_grad_(False)
|
||
# text_encoder.text_model.embeddings.token_embedding.requires_grad_(True)
|
||
|
||
unet.requires_grad_(False)
|
||
unet.to(accelerator.device, dtype=weight_dtype)
|
||
if args.gradient_checkpointing: # according to TI example in Diffusers, train is required
|
||
unet.train()
|
||
else:
|
||
unet.eval()
|
||
|
||
if not cache_latents:
|
||
vae.requires_grad_(False)
|
||
vae.eval()
|
||
vae.to(accelerator.device, dtype=weight_dtype)
|
||
|
||
# 実験的機能:勾配も含めたfp16学習を行う PyTorchにパッチを当ててfp16でのgrad scaleを有効にする
|
||
if args.full_fp16:
|
||
train_util.patch_accelerator_for_fp16_training(accelerator)
|
||
text_encoder.to(weight_dtype)
|
||
|
||
# resumeする
|
||
if args.resume is not None:
|
||
print(f"resume training from state: {args.resume}")
|
||
accelerator.load_state(args.resume)
|
||
|
||
# epoch数を計算する
|
||
num_update_steps_per_epoch = math.ceil(len(train_dataloader) / args.gradient_accumulation_steps)
|
||
num_train_epochs = math.ceil(args.max_train_steps / num_update_steps_per_epoch)
|
||
if (args.save_n_epoch_ratio is not None) and (args.save_n_epoch_ratio > 0):
|
||
args.save_every_n_epochs = math.floor(num_train_epochs / args.save_n_epoch_ratio) or 1
|
||
|
||
# 学習する
|
||
total_batch_size = args.train_batch_size * accelerator.num_processes * args.gradient_accumulation_steps
|
||
print("running training / 学習開始")
|
||
print(f" num train images * repeats / 学習画像の数×繰り返し回数: {train_dataset.num_train_images}")
|
||
print(f" num reg images / 正則化画像の数: {train_dataset.num_reg_images}")
|
||
print(f" num batches per epoch / 1epochのバッチ数: {len(train_dataloader)}")
|
||
print(f" num epochs / epoch数: {num_train_epochs}")
|
||
print(f" batch size per device / バッチサイズ: {args.train_batch_size}")
|
||
print(f" total train batch size (with parallel & distributed & accumulation) / 総バッチサイズ(並列学習、勾配合計含む): {total_batch_size}")
|
||
print(f" gradient ccumulation steps / 勾配を合計するステップ数 = {args.gradient_accumulation_steps}")
|
||
print(f" total optimization steps / 学習ステップ数: {args.max_train_steps}")
|
||
|
||
progress_bar = tqdm(range(args.max_train_steps), smoothing=0, disable=not accelerator.is_local_main_process, desc="steps")
|
||
global_step = 0
|
||
|
||
noise_scheduler = DDPMScheduler(beta_start=0.00085, beta_end=0.012, beta_schedule="scaled_linear",
|
||
num_train_timesteps=1000, clip_sample=False)
|
||
|
||
if accelerator.is_main_process:
|
||
accelerator.init_trackers("textual_inversion")
|
||
|
||
for epoch in range(num_train_epochs):
|
||
print(f"epoch {epoch+1}/{num_train_epochs}")
|
||
train_dataset.set_current_epoch(epoch + 1)
|
||
|
||
text_encoder.train()
|
||
|
||
loss_total = 0
|
||
bef_epo_embs = unwrap_model(text_encoder).get_input_embeddings().weight[token_ids].data.detach().clone()
|
||
for step, batch in enumerate(train_dataloader):
|
||
with accelerator.accumulate(text_encoder):
|
||
with torch.no_grad():
|
||
if "latents" in batch and batch["latents"] is not None:
|
||
latents = batch["latents"].to(accelerator.device)
|
||
else:
|
||
# latentに変換
|
||
latents = vae.encode(batch["images"].to(dtype=weight_dtype)).latent_dist.sample()
|
||
latents = latents * 0.18215
|
||
b_size = latents.shape[0]
|
||
|
||
# Get the text embedding for conditioning
|
||
input_ids = batch["input_ids"].to(accelerator.device)
|
||
# weight_dtype) use float instead of fp16/bf16 because text encoder is float
|
||
encoder_hidden_states = train_util.get_hidden_states(args, input_ids, tokenizer, text_encoder, torch.float)
|
||
|
||
# Sample noise that we'll add to the latents
|
||
noise = torch.randn_like(latents, device=latents.device)
|
||
|
||
# Sample a random timestep for each image
|
||
timesteps = torch.randint(0, noise_scheduler.config.num_train_timesteps, (b_size,), device=latents.device)
|
||
timesteps = timesteps.long()
|
||
|
||
# Add noise to the latents according to the noise magnitude at each timestep
|
||
# (this is the forward diffusion process)
|
||
noisy_latents = noise_scheduler.add_noise(latents, noise, timesteps)
|
||
|
||
# Predict the noise residual
|
||
noise_pred = unet(noisy_latents, timesteps, encoder_hidden_states).sample
|
||
|
||
if args.v_parameterization:
|
||
# v-parameterization training
|
||
target = noise_scheduler.get_velocity(latents, noise, timesteps)
|
||
else:
|
||
target = noise
|
||
|
||
loss = torch.nn.functional.mse_loss(noise_pred.float(), target.float(), reduction="none")
|
||
loss = loss.mean([1, 2, 3])
|
||
|
||
loss_weights = batch["loss_weights"] # 各sampleごとのweight
|
||
loss = loss * loss_weights
|
||
|
||
loss = loss.mean() # 平均なのでbatch_sizeで割る必要なし
|
||
|
||
accelerator.backward(loss)
|
||
if accelerator.sync_gradients:
|
||
params_to_clip = text_encoder.get_input_embeddings().parameters()
|
||
accelerator.clip_grad_norm_(params_to_clip, 1.0) # args.max_grad_norm)
|
||
|
||
optimizer.step()
|
||
lr_scheduler.step()
|
||
optimizer.zero_grad(set_to_none=True)
|
||
|
||
# Let's make sure we don't update any embedding weights besides the newly added token
|
||
with torch.no_grad():
|
||
unwrap_model(text_encoder).get_input_embeddings().weight[index_no_updates] = orig_embeds_params[index_no_updates]
|
||
|
||
# Checks if the accelerator has performed an optimization step behind the scenes
|
||
if accelerator.sync_gradients:
|
||
progress_bar.update(1)
|
||
global_step += 1
|
||
|
||
current_loss = loss.detach().item()
|
||
if args.logging_dir is not None:
|
||
logs = {"loss": current_loss, "lr": lr_scheduler.get_last_lr()[0]}
|
||
accelerator.log(logs, step=global_step)
|
||
|
||
loss_total += current_loss
|
||
avr_loss = loss_total / (step+1)
|
||
logs = {"loss": avr_loss} # , "lr": lr_scheduler.get_last_lr()[0]}
|
||
progress_bar.set_postfix(**logs)
|
||
|
||
if global_step >= args.max_train_steps:
|
||
break
|
||
|
||
if args.logging_dir is not None:
|
||
logs = {"loss/epoch": loss_total / len(train_dataloader)}
|
||
accelerator.log(logs, step=epoch+1)
|
||
|
||
accelerator.wait_for_everyone()
|
||
|
||
updated_embs = unwrap_model(text_encoder).get_input_embeddings().weight[token_ids].data.detach().clone()
|
||
# d = updated_embs - bef_epo_embs
|
||
# print(bef_epo_embs.size(), updated_embs.size(), d.mean(), d.min())
|
||
|
||
if args.save_every_n_epochs is not None:
|
||
model_name = train_util.DEFAULT_EPOCH_NAME if args.output_name is None else args.output_name
|
||
|
||
def save_func():
|
||
ckpt_name = train_util.EPOCH_FILE_NAME.format(model_name, epoch + 1) + '.' + args.save_model_as
|
||
ckpt_file = os.path.join(args.output_dir, ckpt_name)
|
||
print(f"saving checkpoint: {ckpt_file}")
|
||
save_weights(ckpt_file, updated_embs, save_dtype)
|
||
|
||
def remove_old_func(old_epoch_no):
|
||
old_ckpt_name = train_util.EPOCH_FILE_NAME.format(model_name, old_epoch_no) + '.' + args.save_model_as
|
||
old_ckpt_file = os.path.join(args.output_dir, old_ckpt_name)
|
||
if os.path.exists(old_ckpt_file):
|
||
print(f"removing old checkpoint: {old_ckpt_file}")
|
||
os.remove(old_ckpt_file)
|
||
|
||
saving = train_util.save_on_epoch_end(args, save_func, remove_old_func, epoch + 1, num_train_epochs)
|
||
if saving and args.save_state:
|
||
train_util.save_state_on_epoch_end(args, accelerator, model_name, epoch + 1)
|
||
|
||
# end of epoch
|
||
|
||
is_main_process = accelerator.is_main_process
|
||
if is_main_process:
|
||
text_encoder = unwrap_model(text_encoder)
|
||
|
||
accelerator.end_training()
|
||
|
||
if args.save_state:
|
||
train_util.save_state_on_train_end(args, accelerator)
|
||
|
||
updated_embs = text_encoder.get_input_embeddings().weight[token_ids].data.detach().clone()
|
||
|
||
del accelerator # この後メモリを使うのでこれは消す
|
||
|
||
if is_main_process:
|
||
os.makedirs(args.output_dir, exist_ok=True)
|
||
|
||
model_name = train_util.DEFAULT_LAST_OUTPUT_NAME if args.output_name is None else args.output_name
|
||
ckpt_name = model_name + '.' + args.save_model_as
|
||
ckpt_file = os.path.join(args.output_dir, ckpt_name)
|
||
|
||
print(f"save trained model to {ckpt_file}")
|
||
save_weights(ckpt_file, updated_embs, save_dtype)
|
||
print("model saved.")
|
||
|
||
|
||
def save_weights(file, updated_embs, save_dtype):
|
||
state_dict = {"emb_params": updated_embs}
|
||
|
||
if save_dtype is not None:
|
||
for key in list(state_dict.keys()):
|
||
v = state_dict[key]
|
||
v = v.detach().clone().to("cpu").to(save_dtype)
|
||
state_dict[key] = v
|
||
|
||
if os.path.splitext(file)[1] == '.safetensors':
|
||
from safetensors.torch import save_file
|
||
save_file(state_dict, file)
|
||
else:
|
||
torch.save(state_dict, file) # can be loaded in Web UI
|
||
|
||
|
||
def load_weights(file):
|
||
if os.path.splitext(file)[1] == '.safetensors':
|
||
from safetensors.torch import load_file
|
||
data = load_file(file)
|
||
else:
|
||
# compatible to Web UI's file format
|
||
data = torch.load(file, map_location='cpu')
|
||
if type(data) != dict:
|
||
raise ValueError(f"weight file is not dict / 重みファイルがdict形式ではありません: {file}")
|
||
|
||
if 'string_to_param' in data: # textual inversion embeddings
|
||
data = data['string_to_param']
|
||
if hasattr(data, '_parameters'): # support old PyTorch?
|
||
data = getattr(data, '_parameters')
|
||
|
||
emb = next(iter(data.values()))
|
||
if type(emb) != torch.Tensor:
|
||
raise ValueError(f"weight file does not contains Tensor / 重みファイルのデータがTensorではありません: {file}")
|
||
|
||
if len(emb.size()) == 1:
|
||
emb = emb.unsqueeze(0)
|
||
|
||
return emb
|
||
|
||
|
||
if __name__ == '__main__':
|
||
parser = argparse.ArgumentParser()
|
||
|
||
train_util.add_sd_models_arguments(parser)
|
||
train_util.add_dataset_arguments(parser, True, True, False)
|
||
train_util.add_training_arguments(parser, True)
|
||
|
||
parser.add_argument("--save_model_as", type=str, default="pt", choices=[None, "ckpt", "pt", "safetensors"],
|
||
help="format to save the model (default is .pt) / モデル保存時の形式(デフォルトはpt)")
|
||
|
||
parser.add_argument("--weights", type=str, default=None,
|
||
help="embedding weights to initialize / 学習するネットワークの初期重み")
|
||
parser.add_argument("--num_vectors_per_token", type=int, default=1,
|
||
help='number of vectors per token / トークンに割り当てるembeddingsの要素数')
|
||
parser.add_argument("--token_string", type=str, default=None,
|
||
help="token string used in training, must not exist in tokenizer / 学習時に使用されるトークン文字列、tokenizerに存在しない文字であること")
|
||
parser.add_argument("--init_word", type=str, default=None,
|
||
help="words to initialize vector / ベクトルを初期化に使用する単語、複数可")
|
||
parser.add_argument("--use_object_template", action='store_true',
|
||
help="ignore caption and use default templates for object / キャプションは使わずデフォルトの物体用テンプレートで学習する")
|
||
parser.add_argument("--use_style_template", action='store_true',
|
||
help="ignore caption and use default templates for stype / キャプションは使わずデフォルトのスタイル用テンプレートで学習する")
|
||
|
||
args = parser.parse_args()
|
||
train(args)
|